6. Validation
Let’s go through an end-to-end process in training and validating a classification model. After understanding this process, you may modify it for your own purpose. In general, the steps are as follows.
Data: you need to acquire your data relevant to the problem and goal you are trying to achieve; here we generate synthetic data.
Missingness: typically, there is missing data and we need to figure a way to resolve this issue; here, we randomly force data to be missing at random.
Splitting: when we train a model, we desire for the model to be able to
generalize
into the future againstunseen
data; here, we split the data into training and validation sets, where the modeling pipeline will only see the training data and will be tested for performance against the unseen validation set.Training: the training pipeline is dependent on the nature and properties of your data; here, our pipeline is one-hot encoding, imputation, scaling and finally, training a model.
Validation: after we done training a model, we cross-validate the model against unseen data; here, the unseen data is the holdout validation set.
6.1. Generate data
Below, we simulate data D
.
\(X_1 \sim \mathcal{N}(0, 1)\)
\(X_2 \sim \mathcal{N}(0, 1)\)
\(X_3\) is
red
when \(X_1 < 0\) and \(X_2 < 0\)green
when \(X_1 > 0\) and \(X_2 > 0\)blue
otherwise
\(Y_0 = 1 + 2X_1 + 3X_2 + \sigma\)
\(Y_1 = \frac{1}{1 + \exp(-Y_0)}\)
\(y \sim \mathcal{Binom}(Y_1)\)
[1]:
suppressMessages({
library('dplyr')
library('purrr')
})
set.seed(37)
getColor <- function(a, b) {
if (a < 0 && b < 0) {
return('red')
} else if (a > 0 && b > 0) {
return('green')
} else {
return('blue')
}
}
getData <- function(N=1000) {
x1 <- rnorm(N, mean=0, sd=1)
x2 <- rnorm(N, mean=0, sd=1)
x3 <- map2(x1, x2, getColor) %>% flatten_chr()
y <- 1 + 2.0 * x1 + 3.0 * x2 + rnorm(N, mean=0, sd=1)
y <- 1.0 / (1.0 + exp(-y))
y <- rbinom(n=N, size=1, prob=y)
df <- data.frame(x1=x1, x2=x2, x3=x3, y=y)
df <- df %>%
mutate(y=ifelse(y == 0, 'neg', 'pos')) %>%
mutate_at('y', as.factor)
return(df)
}
D <- getData()
[2]:
print(sapply(D, is.factor))
x1 x2 x3 y
FALSE FALSE TRUE TRUE
[3]:
print(head(D))
x1 x2 x3 y
1 0.1247540 0.4817417 green pos
2 0.3820746 0.8176848 green pos
3 0.5792428 -0.3254274 blue pos
4 -0.2937481 -0.2674061 red pos
5 -0.8283492 1.7163252 blue pos
6 -0.3327136 1.2376945 blue pos
[4]:
print(summary(D))
x1 x2 x3 y
Min. :-2.8613 Min. :-3.28763 blue :502 neg:394
1st Qu.:-0.6961 1st Qu.:-0.59550 green:257 pos:606
Median :-0.0339 Median : 0.06348 red :241
Mean :-0.0184 Mean : 0.03492
3rd Qu.: 0.6836 3rd Qu.: 0.69935
Max. : 3.8147 Max. : 3.17901
[5]:
print(dim(D))
[1] 1000 4
6.2. Generate missing data
Here, we make 10% of the data missing in D
and store the results in D.M
.
[6]:
suppressMessages({
library('missForest')
})
D.M <- prodNA(D[c('x1', 'x2', 'x3')], noNA=0.1)
D.M$y <- D$y
[7]:
print(summary(D.M))
x1 x2 x3 y
Min. :-2.86131 Min. :-3.28763 blue :457 neg:394
1st Qu.:-0.70096 1st Qu.:-0.61616 green:225 pos:606
Median :-0.02752 Median : 0.06288 red :213
Mean :-0.03416 Mean : 0.03213 NA's :105
3rd Qu.: 0.65422 3rd Qu.: 0.69815
Max. : 3.81473 Max. : 3.17901
NA's :102 NA's :93
[8]:
print(dim(D))
[1] 1000 4
6.3. Split data
Now we split D.M
into 80% training D.T
and 20% testing D.V
.
[9]:
suppressMessages({
library('caret')
})
trIndices <- createDataPartition(D$y, times=1, p=0.8, list=FALSE)
D.T <- D.M[trIndices, ]
D.V <- D.M[-trIndices, ]
[10]:
print(head(D.T))
x1 x2 x3 y
1 0.1247540 0.4817417 green pos
2 0.3820746 0.8176848 green pos
3 0.5792428 -0.3254274 blue pos
5 NA 1.7163252 blue pos
7 -0.1921595 -1.1223299 red neg
8 1.3629827 1.5202646 <NA> pos
[11]:
print(head(D.V))
x1 x2 x3 y
4 -0.2937481 -0.2674061 red pos
6 -0.3327136 1.2376945 blue pos
26 -0.4590474 1.5723978 blue pos
29 -2.1039691 1.0775911 blue pos
33 0.6553955 NA blue neg
36 0.1297151 0.2434078 green pos
[12]:
print(dim(D.T))
[1] 801 4
[13]:
print(dim(D.V))
[1] 199 4
6.4. Profile data
6.4.1. Statistical profiles
Now, let’s quickly look at the data profiles.
6.4.1.1. Training profile
[14]:
library('skimr')
skimmed <- skim(D.T[,1:2])
s <- skimmed[, c(2:6, 12)]
print(s)
# A tibble: 2 x 6
skim_variable n_missing complete_rate numeric.mean numeric.sd numeric.hist
<chr> <int> <dbl> <dbl> <dbl> <chr>
1 x1 87 0.891 -0.0156 0.994 ▁▅▇▃▁
2 x2 73 0.909 0.0337 1.04 ▁▃▇▅▁
[15]:
skimmed <- skim(D.T[,3:4])
s <- skimmed[, c(2:7)]
print(s)
# A tibble: 2 x 6
skim_variable n_missing complete_rate factor.ordered factor.n_unique
<chr> <int> <dbl> <lgl> <int>
1 x3 81 0.899 FALSE 3
2 y 0 1 FALSE 2
# … with 1 more variable: factor.top_counts <chr>
6.4.1.2. Validation profile
[16]:
skimmed <- skim(D.V[,1:2])
s <- skimmed[, c(2:6, 12)]
print(s)
# A tibble: 2 x 6
skim_variable n_missing complete_rate numeric.mean numeric.sd numeric.hist
<chr> <int> <dbl> <dbl> <dbl> <chr>
1 x1 15 0.925 -0.106 1.05 ▂▇▇▂▁
2 x2 20 0.899 0.0257 0.977 ▁▂▇▅▁
[17]:
skimmed <- skim(D.V[,3:4])
s <- skimmed[, c(2:7)]
print(s)
# A tibble: 2 x 6
skim_variable n_missing complete_rate factor.ordered factor.n_unique
<chr> <int> <dbl> <lgl> <int>
1 x3 24 0.879 FALSE 3
2 y 0 1 FALSE 2
# … with 1 more variable: factor.top_counts <chr>
6.4.2. Missingness profiles
Below, we visually inspect the data missingness.
[18]:
suppressMessages({
library('VIM')
})
options(repr.plot.width=4, repr.plot.height=4)
p <- aggr(
D.M,
col=c('navyblue','yellow'),
numbers=TRUE,
sortVars=TRUE,
labels=names(D.M),
cex.axis=.7,
gap=3,
ylab=c('Missing data', 'Pattern')
)
Variables sorted by number of missings:
Variable Count
x3 0.105
x1 0.102
x2 0.093
y 0.000
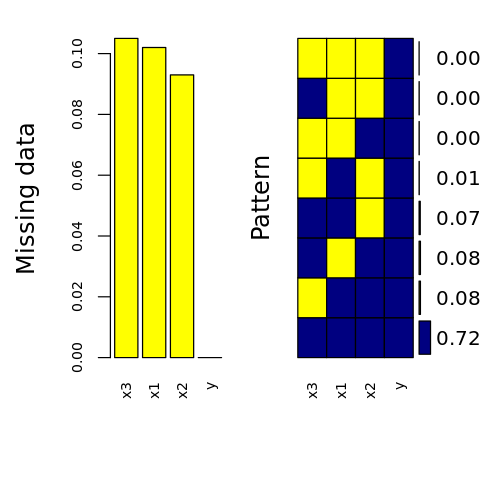
[19]:
p <- aggr(
D.T,
col=c('navyblue','yellow'),
numbers=TRUE,
sortVars=TRUE,
labels=names(D.T),
cex.axis=.7,
gap=3,
ylab=c('Missing data', 'Pattern')
)
Warning message in plot.aggr(res, ...):
“not enough horizontal space to display frequencies”
Variables sorted by number of missings:
Variable Count
x1 0.10861423
x3 0.10112360
x2 0.09113608
y 0.00000000
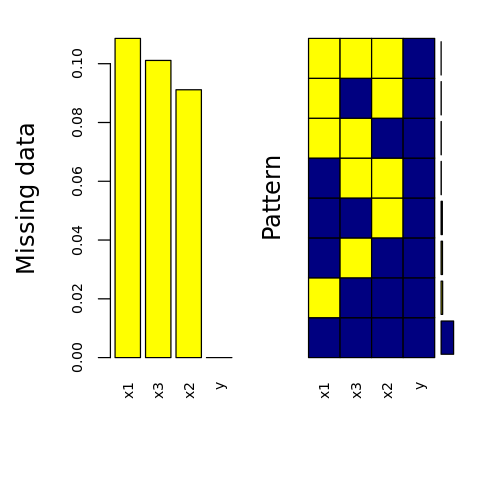
[20]:
p <- aggr(
D.V,
col=c('navyblue','yellow'),
numbers=TRUE,
sortVars=TRUE,
labels=names(D.V),
cex.axis=.7,
gap=3,
ylab=c('Missing data', 'Pattern')
)
Variables sorted by number of missings:
Variable Count
x3 0.12060302
x2 0.10050251
x1 0.07537688
y 0.00000000
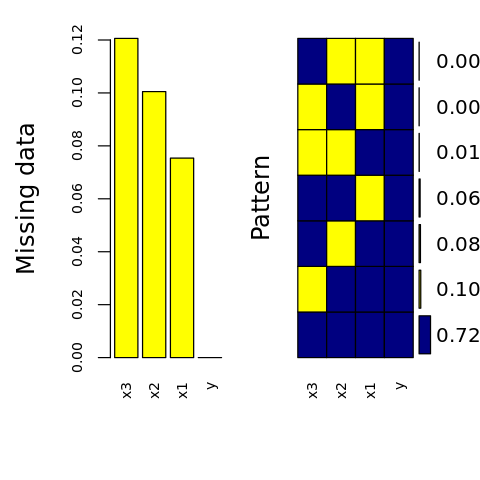
6.5. One-hot encoding
We perform one-hot encoding OHE
on the categorical variables and store the result in D.O
. Note how we start the training pipeline at OHE? The reason is to avoid data leakage
. Had we applied OHE on the whole dataset, we may bias the training process towards better results.
[21]:
ohe <- dummyVars(~ ., data=D.T[,-4], sep='_')
D.O <- data.frame(predict(ohe, newdata=D[,-4]))
D.O$y <- D$y
[22]:
print(head(D.O))
x1 x2 x3_blue x3_green x3_red y
1 0.1247540 0.4817417 0 1 0 pos
2 0.3820746 0.8176848 0 1 0 pos
3 0.5792428 -0.3254274 1 0 0 pos
4 -0.2937481 -0.2674061 0 0 1 pos
5 -0.8283492 1.7163252 1 0 0 pos
6 -0.3327136 1.2376945 1 0 0 pos
[23]:
print(summary(D.O))
x1 x2 x3_blue x3_green
Min. :-2.8613 Min. :-3.28763 Min. :0.000 Min. :0.000
1st Qu.:-0.6961 1st Qu.:-0.59550 1st Qu.:0.000 1st Qu.:0.000
Median :-0.0339 Median : 0.06348 Median :1.000 Median :0.000
Mean :-0.0184 Mean : 0.03492 Mean :0.502 Mean :0.257
3rd Qu.: 0.6836 3rd Qu.: 0.69935 3rd Qu.:1.000 3rd Qu.:1.000
Max. : 3.8147 Max. : 3.17901 Max. :1.000 Max. :1.000
x3_red y
Min. :0.000 neg:394
1st Qu.:0.000 pos:606
Median :0.000
Mean :0.241
3rd Qu.:0.000
Max. :1.000
6.6. Imputation
We will preprocess the training data using k-nearest neighbor
imputation and store the result in D.I
.
[24]:
library('RANN')
imp <- preProcess(D.O, method='knnImpute')
D.I <- predict(imp, newdata=D.O)
print(anyNA(D.I))
[1] FALSE
[25]:
print(head(D.I))
x1 x2 x3_blue x3_green x3_red y
1 0.1408176 0.4391669 -1.0035059 1.6994586 -0.563210 pos
2 0.3939391 0.7693556 -1.0035059 1.6994586 -0.563210 pos
3 0.5878898 -0.3541760 0.9955098 -0.5878343 -0.563210 pos
4 -0.2708553 -0.2971486 -1.0035059 -0.5878343 1.773761 pos
5 -0.7967326 1.6526029 0.9955098 -0.5878343 -0.563210 pos
6 -0.3091849 1.1821708 0.9955098 -0.5878343 -0.563210 pos
[26]:
print(summary(D.I))
x1 x2 x3_blue x3_green
Min. :-2.79652 Min. :-3.26564 Min. :-1.0035 Min. :-0.5878
1st Qu.:-0.66661 1st Qu.:-0.61962 1st Qu.:-1.0035 1st Qu.:-0.5878
Median :-0.01525 Median : 0.02807 Median : 0.9955 Median :-0.5878
Mean : 0.00000 Mean : 0.00000 Mean : 0.0000 Mean : 0.0000
3rd Qu.: 0.69053 3rd Qu.: 0.65305 3rd Qu.: 0.9955 3rd Qu.: 1.6995
Max. : 3.77058 Max. : 3.09023 Max. : 0.9955 Max. : 1.6995
x3_red y
Min. :-0.5632 neg:394
1st Qu.:-0.5632 pos:606
Median :-0.5632
Mean : 0.0000
3rd Qu.:-0.5632
Max. : 1.7738
6.7. Rescale
Let’s rescale the variables into the range [0, 1] and store the result in D.R
.
[27]:
ran <- preProcess(D.I, method='range')
D.R <- predict(ran, newdata=D.I)
[28]:
print(head(D.R))
x1 x2 x3_blue x3_green x3_red y
1 0.4472809 0.5828955 0 1 0 pos
2 0.4858248 0.6348457 0 1 0 pos
3 0.5153584 0.4580750 1 0 0 pos
4 0.3845938 0.4670474 0 0 1 pos
5 0.3045163 0.7738113 1 0 0 pos
6 0.3787572 0.6997959 1 0 0 pos
[29]:
print(summary(D.R))
x1 x2 x3_blue x3_green
Min. :0.0000 Min. :0.0000 Min. :0.000 Min. :0.000
1st Qu.:0.3243 1st Qu.:0.4163 1st Qu.:0.000 1st Qu.:0.000
Median :0.4235 Median :0.5182 Median :1.000 Median :0.000
Mean :0.4258 Mean :0.5138 Mean :0.502 Mean :0.257
3rd Qu.:0.5310 3rd Qu.:0.6165 3rd Qu.:1.000 3rd Qu.:1.000
Max. :1.0000 Max. :1.0000 Max. :1.000 Max. :1.000
x3_red y
Min. :0.000 neg:394
1st Qu.:0.000 pos:606
Median :0.000
Mean :0.241
3rd Qu.:0.000
Max. :1.000
[30]:
print(dim(D.R))
[1] 1000 6
6.8. Feature importance
We may visualize feature importance
to see which features/variables are important to the class variable.
[31]:
featurePlot(x=D.R[,1:5],
y=D.R$y,
plot='box',
strip=strip.custom(par.strip.text=list(cex=.7)),
scales = list(x = list(relation='free'),
y = list(relation='free')))
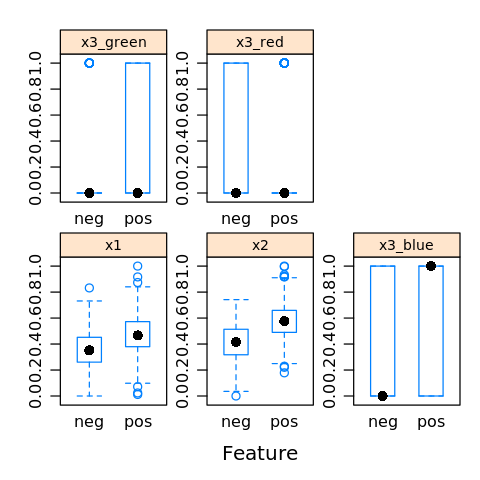
[32]:
featurePlot(x=D.R[,1:5],
y=D.R$y,
plot='density',
strip=strip.custom(par.strip.text=list(cex=.7)),
scales = list(x = list(relation='free'),
y = list(relation='free')))
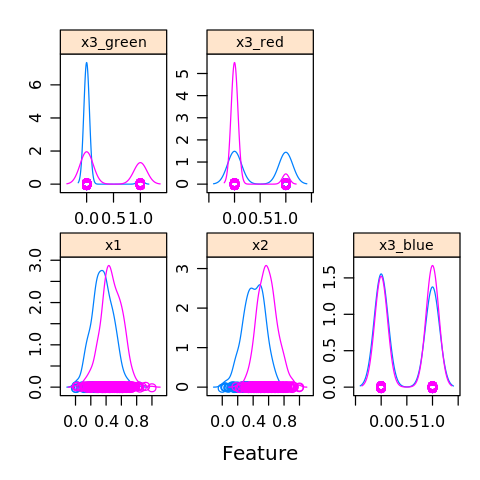
6.9. Feature selection
Feature selection
may help us to use only features (variables) that are important to the classification.
[33]:
ctrl <- rfeControl(
functions=rfFuncs,
method='repeatedcv',
repeats=5,
verbose=FALSE
)
profile <- rfe(y ~ ., data=D.R, sizes=c(2, 3), rfeControl=ctrl)
[34]:
profile
Recursive feature selection
Outer resampling method: Cross-Validated (10 fold, repeated 5 times)
Resampling performance over subset size:
Variables Accuracy Kappa AccuracySD KappaSD Selected
2 0.8028 0.5861 0.03459 0.07315
3 0.8102 0.5908 0.03654 0.08220
5 0.8214 0.6186 0.03640 0.08056 *
The top 5 variables (out of 5):
x2, x1, x3_red, x3_green, x3_blue
6.10. Training
We are now ready to train a classification model. We will use a random forest
to classify the data. Use the modelLookup
function to see which arguments are available. Below, the mtry
argument/parameter is available. The model will be bound to M
.
[35]:
modelLookup('rf')
model | parameter | label | forReg | forClass | probModel |
---|---|---|---|---|---|
<chr> | <fct> | <fct> | <lgl> | <lgl> | <lgl> |
rf | mtry | #Randomly Selected Predictors | TRUE | TRUE | TRUE |
[36]:
M <- train(y ~ ., data=D.R, method='rf')
[37]:
print(M)
Random Forest
1000 samples
5 predictor
2 classes: 'neg', 'pos'
No pre-processing
Resampling: Bootstrapped (25 reps)
Summary of sample sizes: 1000, 1000, 1000, 1000, 1000, 1000, ...
Resampling results across tuning parameters:
mtry Accuracy Kappa
2 0.8192793 0.6111993
3 0.8136866 0.6049471
5 0.7961271 0.5705189
Accuracy was used to select the optimal model using the largest value.
The final value used for the model was mtry = 2.
We may visualize the accuracy
of the training with the predictors varying.
[38]:
plot(M)
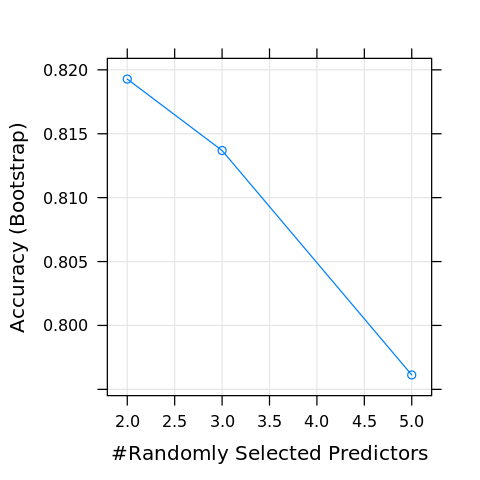
We may visualize the variable importance
.
[39]:
vi <- varImp(M)
plot(vi)
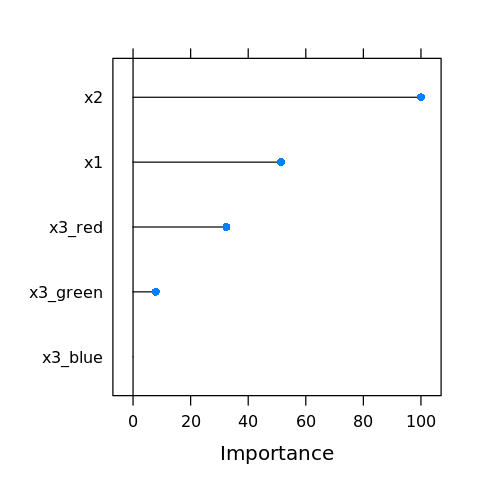
6.11. Predicting on validation data
6.11.1. Transform validation data
We will not run the validation data D.V
through the pipeline.
one-hot encoding,
O
imputation,
I
scaling (to the range [0, 1]),
R
[40]:
O <- predict(ohe, newdata=D.V)
[41]:
I <- predict(imp, newdata=O)
[42]:
R <- predict(ran, newdata=I)
[43]:
print(head(R))
x1 x2 x3_blue x3_green x3_red
4 0.3845938 0.4670474 0 0 1
6 0.3787572 0.6997959 1 0 0
26 0.3598337 0.7515544 1 0 0
29 0.1134421 0.6750376 1 0 0
33 0.5267653 0.4183815 1 0 0
36 0.4480240 0.5460396 0 1 0
[44]:
print(dim(R))
[1] 199 5
6.11.2. Confusion matrix
Let’s look at the confusion matrix
.
[45]:
y <- predict(M, R)
cm <- confusionMatrix(reference=D.V$y, data=y, positive='pos')
[46]:
print(cm)
Confusion Matrix and Statistics
Reference
Prediction neg pos
neg 62 16
pos 16 105
Accuracy : 0.8392
95% CI : (0.7806, 0.8873)
No Information Rate : 0.608
P-Value [Acc > NIR] : 1.118e-12
Kappa : 0.6626
Mcnemar's Test P-Value : 1
Sensitivity : 0.8678
Specificity : 0.7949
Pos Pred Value : 0.8678
Neg Pred Value : 0.7949
Prevalence : 0.6080
Detection Rate : 0.5276
Detection Prevalence : 0.6080
Balanced Accuracy : 0.8313
'Positive' Class : pos
6.11.3. Receiver operating characteristic curve
Let’s look at the area under the curve
(AUC
) for the Receiver Operating Characteristic
curve.
[47]:
library('PRROC')
y <- predict(M, R, type='prob')[,1]
results <- data.frame(
y = D.V$y,
y.pred = y
)
class.0 <- results %>% filter(y == 'neg') %>% select(y.pred)
class.1 <- results %>% filter(y == 'pos') %>% select(y.pred)
[48]:
roc <- roc.curve(
scores.class0=class.0$y.pred,
scores.class1=class.1$y.pred,
curve=TRUE,
rand.compute=TRUE)
plot(roc, legend=1, rand.plot=TRUE)
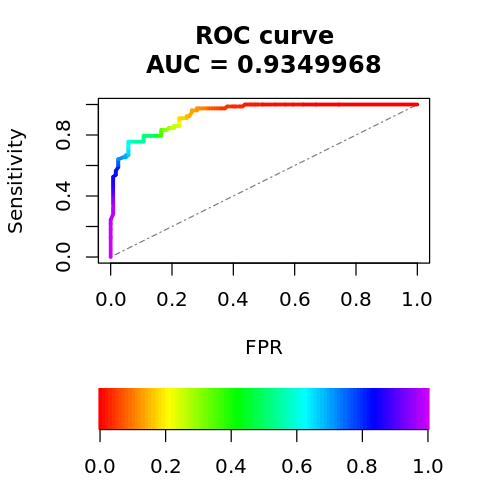
6.11.4. Precision-recall curve
The precision-recall curve is shown below.
[49]:
pr <- pr.curve(
scores.class0=class.0$y.pred,
scores.class1=class.1$y.pred,
curve=TRUE,
rand.compute=TRUE)
plot(pr, legend=1, rand.plot=TRUE)
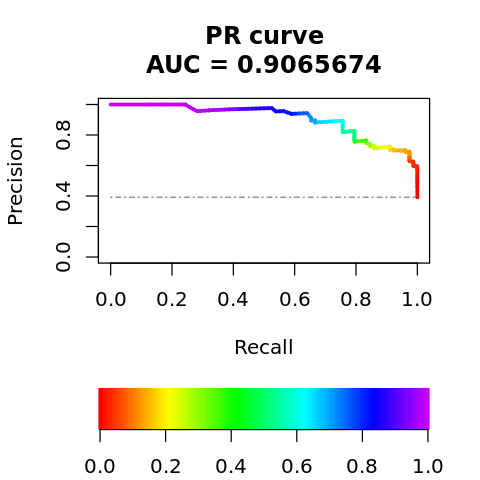