2. ggplot2
The ggplot2
package helps you plot graphics with a grammar
. The grammar provides a way to talk about parts of a plot. The grammar talks about the following components
of a plot.
data
is what is being plottedgeometric objects
are the shapes and lines that appear on the plotaesthetics
are the appearance of the geometric objects and the mapping of variables to such aestheticsposition adjustment
is the placement of elementsscale
is the range of values for each aesthetic mappingcoordinate system
is used to organize the geometric objectsfacets
are groupos of data shown in differen plots
2.1. Geometries
2.1.1. geom_point
The geom_point
is used for drawing individual points.
[1]:
library('ggplot2')
library('repr')
df <- data.frame(
x1 = rnorm(1000),
x2 = rnorm(1000)
)
options(repr.plot.width=4, repr.plot.height=4)
ggplot(df) +
geom_point(mapping=aes(x=x1, y=x2))
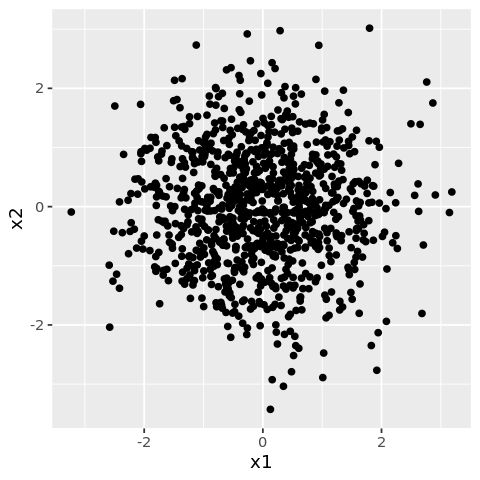
You may also style with the color
attribute.
[2]:
options(repr.plot.width=4, repr.plot.height=4)
ggplot(df) +
geom_point(mapping=aes(x=x1, y=x2), color='firebrick')
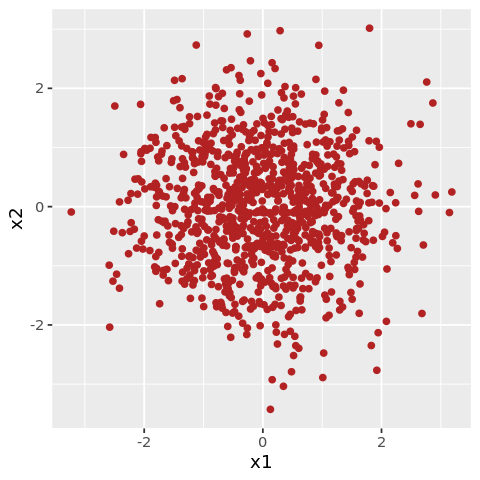
You may also style with themes.
[3]:
library('ggthemes')
options(repr.plot.width=4, repr.plot.height=4)
ggplot(df) +
geom_point(mapping=aes(x=x1, y=x2), color='firebrick') +
theme_economist()
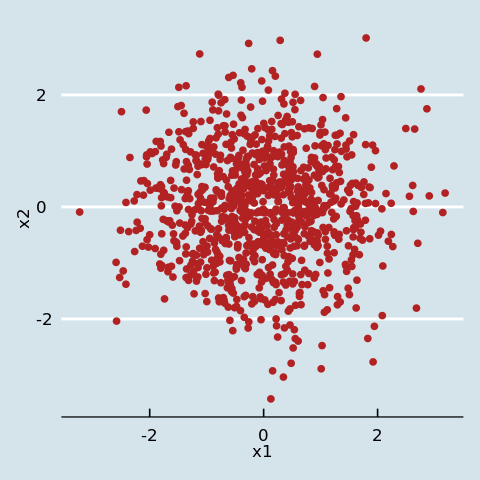
2.1.2. geom_hex
The geom_hex
function is used for drawing individual points as hexagons.
[4]:
df <- data.frame(
x1 = rnorm(1000),
x2 = rnorm(1000)
)
options(repr.plot.width=5, repr.plot.height=4)
ggplot(df) +
geom_hex(mapping=aes(x=x1, y=x2))
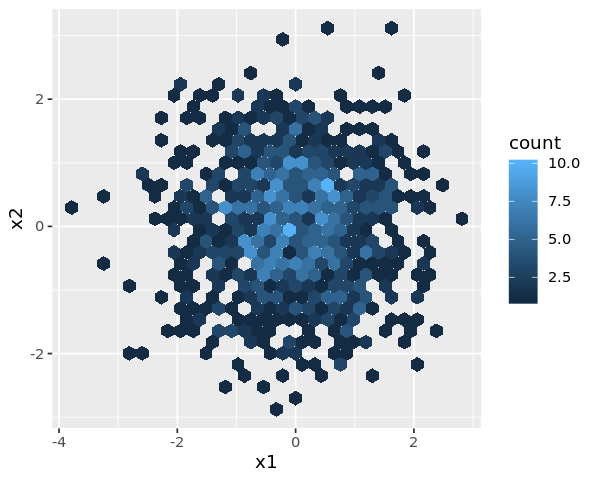
2.1.3. geom_line
The geom_line
function is used for drawing lines.
[5]:
df <- data.frame(
x1 = seq(1, 1000),
x2 = seq(1, 1000)
)
options(repr.plot.width=4, repr.plot.height=4)
ggplot(df) +
geom_line(mapping=aes(x=x1, y=x2))
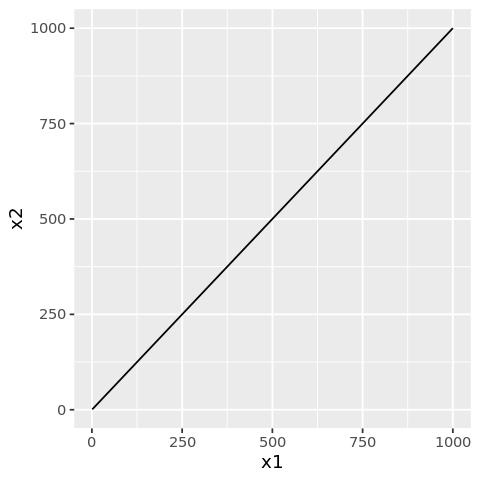
2.1.4. geom_smooth
The geom_smooth
function is used to draw a smoothed line.
[6]:
df <- data.frame(
x1 = seq(1, 1000),
x2 = seq(1, 1000) + (rnorm(1000) * seq(1, 1000))
)
options(repr.plot.width=4, repr.plot.height=4)
ggplot(df) +
geom_point(mapping=aes(x=x1, y=x2)) +
geom_smooth(mapping=aes(x=x1, y=x2))
`geom_smooth()` using method = 'gam' and formula 'y ~ s(x, bs = "cs")'
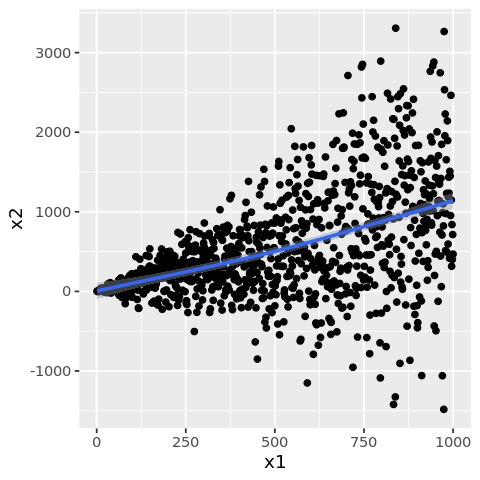
2.1.5. geom_col
The geom_col
function is used to draw bars.
[7]:
df <- data.frame(
x1 = c('DC', 'MD', 'VA'),
x2 = c(20, 30, 15)
)
options(repr.plot.width=4, repr.plot.height=4)
ggplot(df) +
geom_col(mapping=aes(x=x1, y=x2))
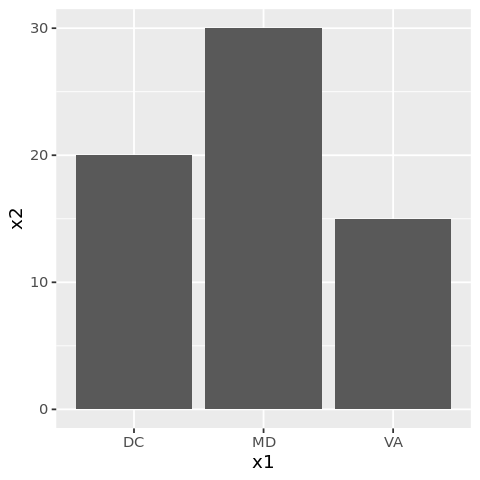
2.1.6. geom_boxplot
Use geom_boxplot
to plot box-whisker plots.
[8]:
df <- data.frame(
x1 = c(rnorm(100, mean=15, sd=5), rnorm(100, mean=25, sd=5)),
x2 = c(rnorm(100, mean=15, sd=5), rnorm(100, mean=25, sd=5)),
state = c(rep('DC', 100), rep('VA', 100))
)
options(repr.plot.width=4, repr.plot.height=4)
ggplot(df) +
geom_boxplot(mapping=aes(x=state, y=x1))
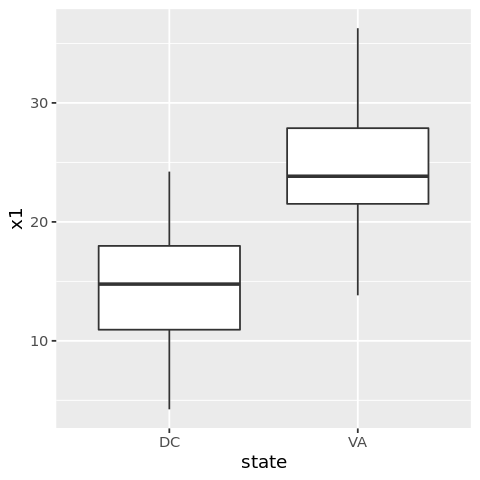
You may play around with attributes to change the look and feel of the box-whisker plot.
[9]:
options(repr.plot.width=5, repr.plot.height=3)
ggplot(df, mapping=aes(x=state, y=x1, color=state)) +
geom_boxplot(
outlier.colour='green',
outlier.shape=8,
notch=TRUE
) +
coord_flip() +
geom_jitter(alpha=0.5, position=position_jitter(width=0.2))
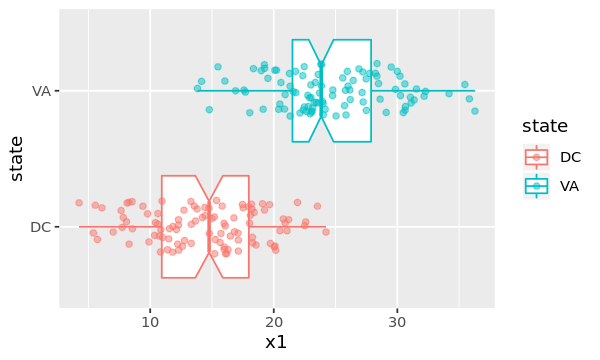
2.1.7. geom_tile
Use geom_tile
to build correlation matrix plots.
[10]:
df <- data.frame(
v1 = c('a', 'a', 'a', 'b', 'b', 'c'),
v2 = c('a', 'b', 'c', 'b', 'c', 'c'),
corr = c(1.0, -0.2, 0.2, 1.0, -1.0, 1.0)
)
options(repr.plot.width=5, repr.plot.height=4)
ggplot(df, mapping=aes(x=v2, y=v1)) +
geom_tile(data=df, aes(fill=corr), color='white') +
scale_fill_gradient2(low='blue', high='red', mid='white', midpoint=0, limit=c(-1, 1)) +
theme(axis.text.x=element_text(angle=45, vjust=1, size=11, hjust=1)) +
coord_equal()
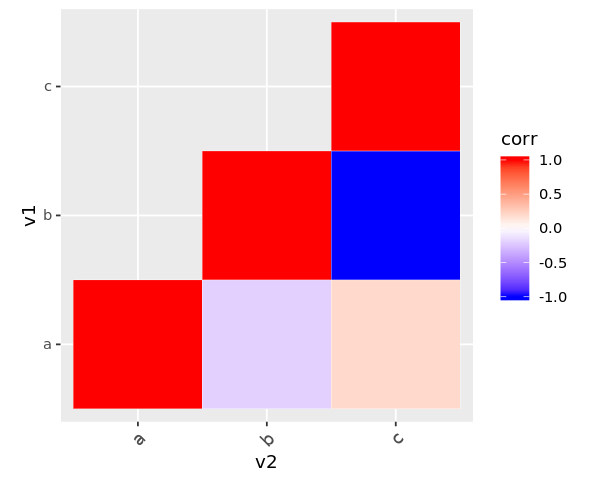
2.2. Aesthetic mapping
Aesthetics may be used to change colors.
[11]:
df <- data.frame(
x1 = c(rnorm(100, mean=1, sd=1), rnorm(100, mean=2.5, sd=1)),
x2 = c(rnorm(100, mean=1, sd=1), rnorm(100, mean=2.5, sd=1)),
state = c(rep('DC', 100), rep('VA', 100))
)
options(repr.plot.width=5, repr.plot.height=4)
ggplot(df) +
geom_point(mapping=aes(x=x1, y=x2, color=state), alpha=0.8)
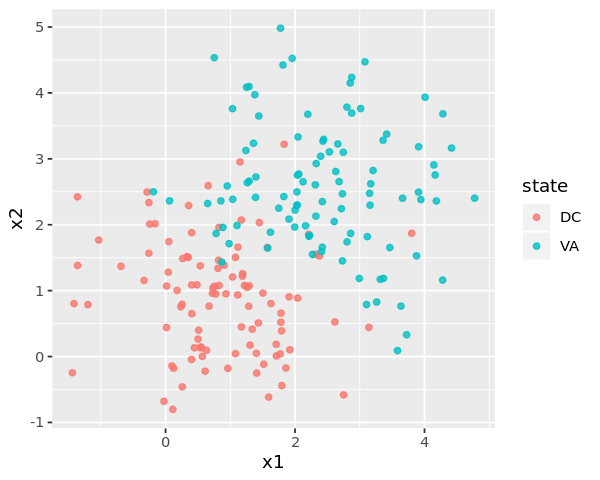
2.3. Position
Positioning can change the placement of elements and produce different types of plots.
[12]:
df <- data.frame(
x1 = c('DC', 'MD', 'VA'),
x2 = c(20, 30, 15)
)
options(repr.plot.width=5, repr.plot.height=4)
ggplot(df) +
geom_col(mapping=aes(x=x1, y=x2, color=x1, fill=x1))
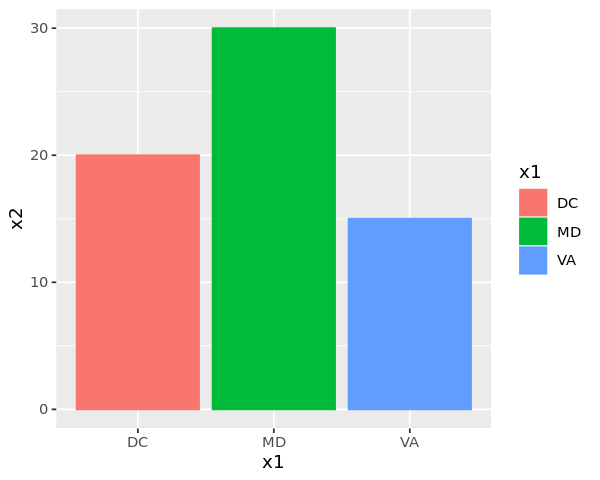
[13]:
library('tidyr')
df <- data.frame(
state = c('DC', 'MD', 'VA'),
water = c(10, 20, 30),
energy = c(12, 25, 39),
social = c(15, 20, 10)
)
n <- df %>%
pivot_longer(-state, names_to='expense', values_to='cost')
options(repr.plot.width=5, repr.plot.height=4)
ggplot(n) +
geom_col(mapping=aes(x=state, y=cost, fill=expense))
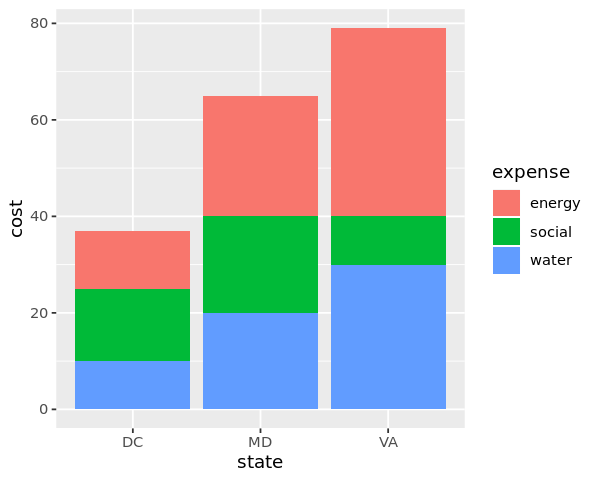
When position='fill'
, the stacked bars are forced to 100%.
[14]:
options(repr.plot.width=5, repr.plot.height=4)
ggplot(n) +
geom_col(mapping=aes(x=state, y=cost, fill=expense), position='fill')
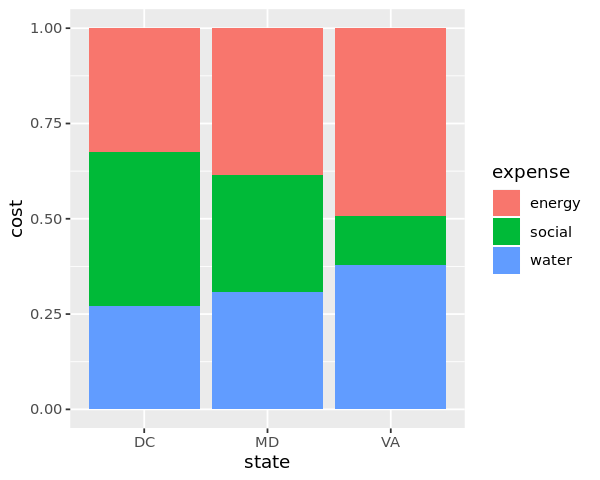
When position='dodge
the bars are side-by-side.
[15]:
options(repr.plot.width=5, repr.plot.height=4)
ggplot(n) +
geom_col(mapping=aes(x=state, y=cost, fill=expense), position='dodge')
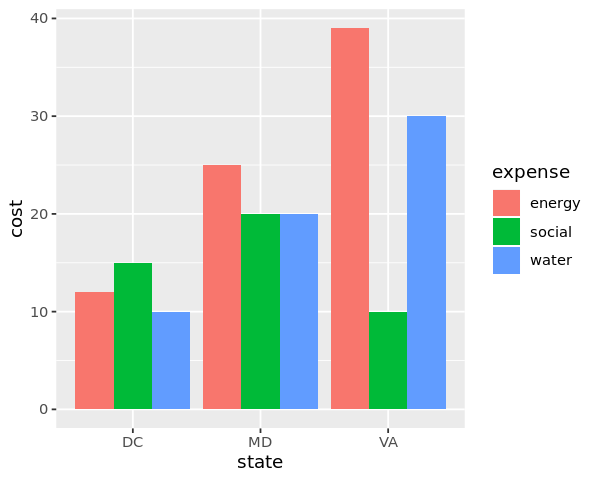
2.4. Scale
Scaling may help to zoom in or out of the plot, as well as rescale the axes.
[16]:
df <- data.frame(
x1 = c(rnorm(100, mean=10, sd=1), rnorm(100, mean=25, sd=1)),
x2 = c(rnorm(100, mean=10, sd=1), rnorm(100, mean=25, sd=1)),
state = c(rep('DC', 100), rep('VA', 100))
)
[17]:
options(repr.plot.width=5, repr.plot.height=4)
ggplot(df) +
geom_point(mapping=aes(x=x1, y=x2, color=state), alpha=0.8) +
scale_color_brewer(palette='Set3') +
scale_x_continuous() +
scale_y_continuous()
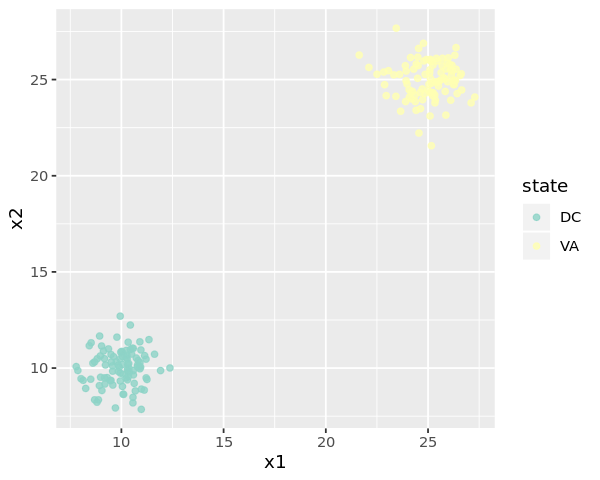
[18]:
options(repr.plot.width=4, repr.plot.height=4)
ggplot(df) +
geom_point(mapping=aes(x=x1, y=x2, color=state), alpha=0.8) +
scale_color_brewer(palette='Set3') +
scale_x_continuous(limits=c(0, 15)) +
scale_y_continuous(limits=c(0, 15)) +
theme(legend.position='none')
Warning message:
“Removed 100 rows containing missing values (geom_point).”
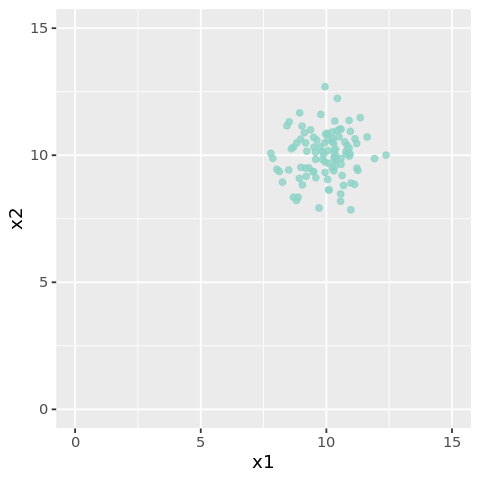
[19]:
options(repr.plot.width=4, repr.plot.height=4)
ggplot(df) +
geom_point(mapping=aes(x=x1, y=x2, color=state), alpha=0.8) +
scale_color_brewer(palette='Set3') +
scale_x_continuous(limits=c(20, 30)) +
scale_y_continuous(limits=c(20, 30)) +
theme(legend.position='none')
Warning message:
“Removed 100 rows containing missing values (geom_point).”
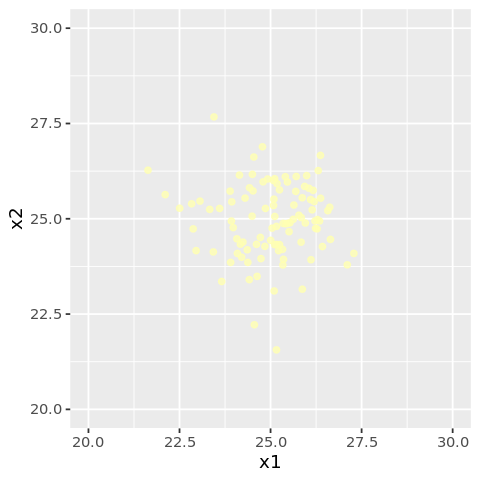
[20]:
options(repr.plot.width=5, repr.plot.height=4)
ggplot(df) +
geom_point(mapping=aes(x=x1, y=x2, color=state), alpha=0.8) +
scale_color_brewer(palette='Set3') +
scale_x_log10() +
scale_y_log10()
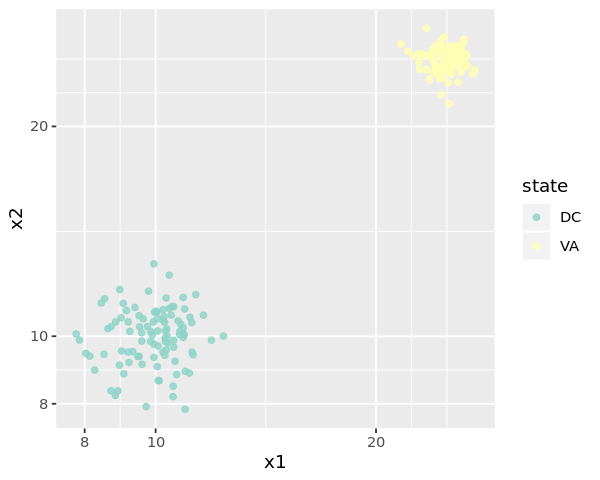
2.5. Coordinate
Coordinate functions such as coord_flip
and coord_polar
can change the type and look of a plot.
[21]:
df <- data.frame(
state = c('DC', 'MD', 'VA'),
water = c(10, 20, 30),
energy = c(12, 25, 39),
social = c(15, 20, 10)
)
n <- df %>%
pivot_longer(-state, names_to='expense', values_to='cost')
options(repr.plot.width=5, repr.plot.height=4)
ggplot(n) +
geom_col(mapping=aes(x=state, y=cost, fill=expense), position='dodge') +
coord_flip()
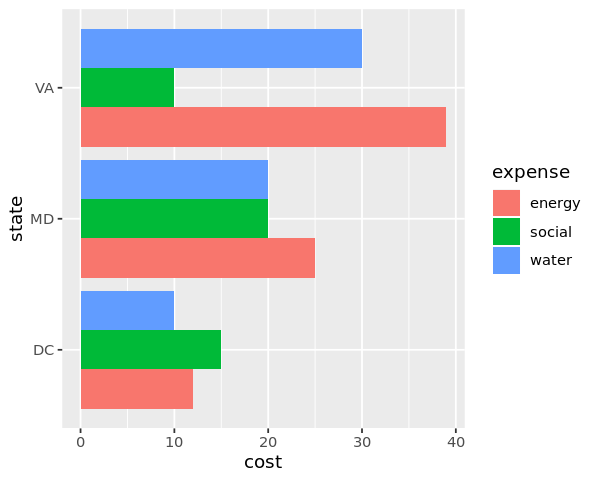
[22]:
options(repr.plot.width=5, repr.plot.height=4)
ggplot(n) +
geom_col(mapping=aes(x=state, y=cost, fill=expense), position='dodge') +
coord_polar()
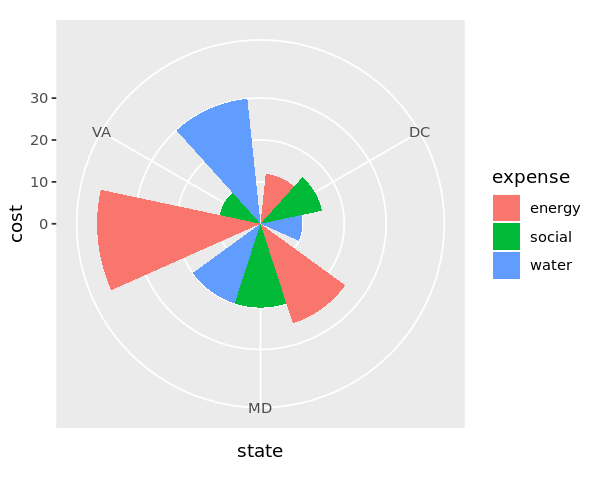
2.6. Facets
Facets can create subplots.
[23]:
df <- data.frame(
state = c('DC', 'MD', 'VA'),
water = c(10, 20, 30),
energy = c(12, 25, 39),
social = c(15, 20, 10)
)
n <- df %>%
pivot_longer(-state, names_to='expense', values_to='cost')
options(repr.plot.width=7, repr.plot.height=3)
ggplot(n) +
geom_col(mapping=aes(x=expense, y=cost, fill=expense)) +
facet_wrap(~state) +
theme(legend.position='none')
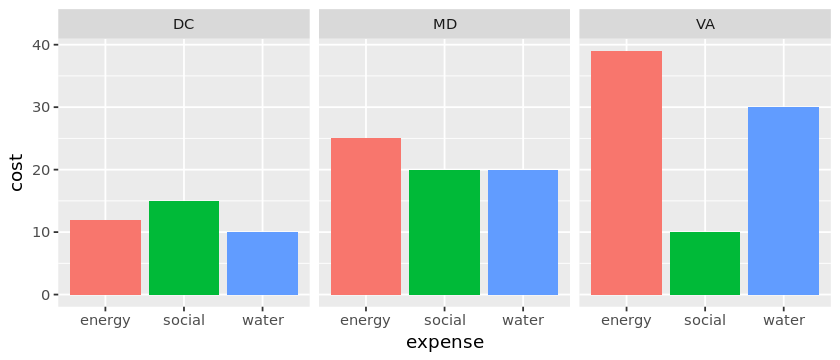
2.7. Labels and annotations
You may modify the title, axes and legend with labs
. You may use geom_label_repel
from the ggrepel
library to annotate specific coordinates.
[24]:
library('ggrepel')
df <- data.frame(
x1 = c(rnorm(100, mean=1, sd=1), rnorm(100, mean=2.5, sd=1)),
x2 = c(rnorm(100, mean=1, sd=1), rnorm(100, mean=2.5, sd=1)),
state = c(rep('DC', 100), rep('VA', 100))
)
centers <- data.frame(
x1 = c(1.0, 2.5),
x2 = c(1.0, 2.5),
state = c('DC', 'VA')
)
options(repr.plot.width=5, repr.plot.height=4)
ggplot(df) +
geom_point(mapping=aes(x=x1, y=x2, color=state), alpha=0.8) +
geom_label_repel(data=centers, mapping=aes(x=x1, y=x2, label=state)) +
labs(
title ='the-title',
x='x-axis',
y='y-axis',
color='the-legend'
) +
theme(
plot.title=element_text(
size=15,
face='bold',
margin=margin(10, 0, 10, 0),
vjust=1,
family='Times'
),
plot.background=element_rect(fill='grey')
)
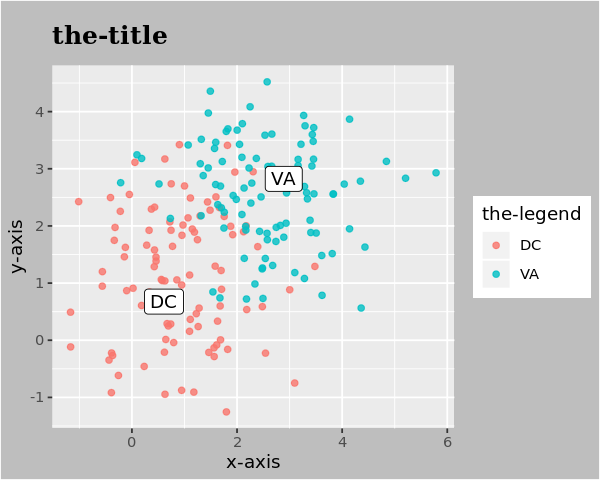
2.8. Choropleth map
[25]:
suppressMessages({
library('dplyr')
})
allStates <- unique(map_data('state')$region)
x <- rnorm(length(allStates), mean=1, sd=1)
randomData <- data.frame(
region=allStates,
weight=x,
stringsAsFactors=FALSE
)
df <- randomData %>%
left_join(map_data('state'), by='region')
ggplot(df) +
geom_polygon(
mapping=aes(x=long, y=lat, group=group, fill=weight),
color='white',
size=0.1
) +
coord_map() +
scale_fill_continuous(low='#132B43', high='Red')
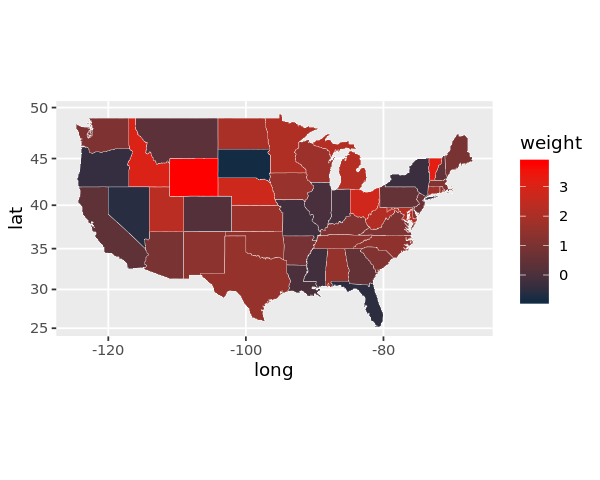
2.9. Subplots
Use the grid
package.
[26]:
suppressMessages({
library('grid')
library('gridExtra')
})
allStates <- unique(map_data('state')$region)
randomData1 <- data.frame(
region=allStates,
weight=rnorm(length(allStates), mean=1, sd=1),
stringsAsFactors=FALSE
)
randomData2 <- data.frame(
region=allStates,
weight=rnorm(length(allStates), mean=10, sd=2),
stringsAsFactors=FALSE
)
df1 <- randomData1 %>%
left_join(map_data('state'), by='region')
df2 <- randomData2 %>%
left_join(map_data('state'), by='region')
plt1 <- ggplot(df1) +
geom_polygon(
mapping=aes(x=long, y=lat, group=group, fill=weight),
color='white',
size=0.1
) +
coord_map() +
scale_fill_continuous(low='#132B43', high='Red') +
theme(legend.position='none')
plt2 <- ggplot(df2) +
geom_polygon(
mapping=aes(x=long, y=lat, group=group, fill=weight),
color='white',
size=0.1
) +
coord_map() +
scale_fill_continuous(low='#132B43', high='Blue') +
theme(legend.position='none')
options(repr.plot.width=10, repr.plot.height=4)
grid.arrange(plt1, plt2, ncol=2)
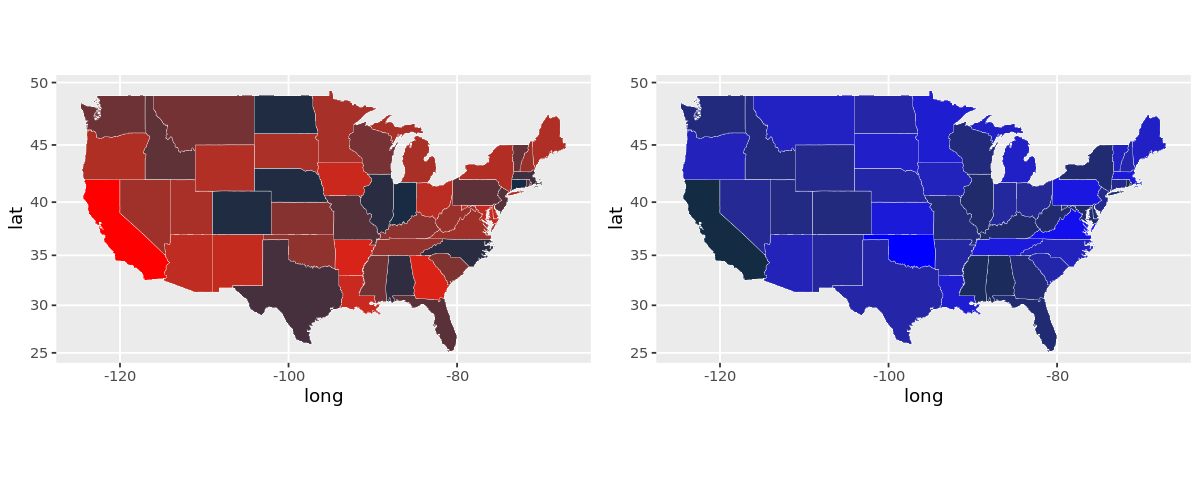